Working of Different Types of Data Analytics
Introduction
With the exponential growth of data over the years, it has become a necessity analyse the data. This allows to discover patterns within the data, understand the trends and help to strategize the approach moving forward. It is must for staying competitive. Understanding the past performance using the historical data, or predicting what’s going to happen, or prescribing the best step forward, all these benefits that data analytics and its types plays an important role for shaping strategies and results. Delving onto these thoughts, this blog covers how different types of data analytics work – Descriptive, Diagnostic, Predictive and Prescriptive.
Before understanding how exactly these types work, visit our previous blog to understand what they are, examples and use cases. Click on the link – Introduction to Data Analytics
How does the different Data Analytics work?
Now, let’s understand how the different types of data analytics work.
Descriptive Analytics
Analysing historical data for comprehending past performance and revealing trends, patterns, and insights is the essential aim of descriptive analytics. Several sources are cited for gathering data, which is the first step in the process, which is then cleaned and pre-processed to guarantee accuracy and reliability. Using summary statistics like mean, median, and standard deviation for concise overviews, the data is visually examined by the analysts to find the trends, outliers, and features. Dashboards, line graphs, and bar charts, etc are examples of data visualization approaches that are really useful in efficiently communicating insights to the stakeholders. Companies can evaluate performance, spot opportunities, and increase efficiency by using time series analysis or segmentation to find patterns, differences, or similarities. This is how this type of analytics works.
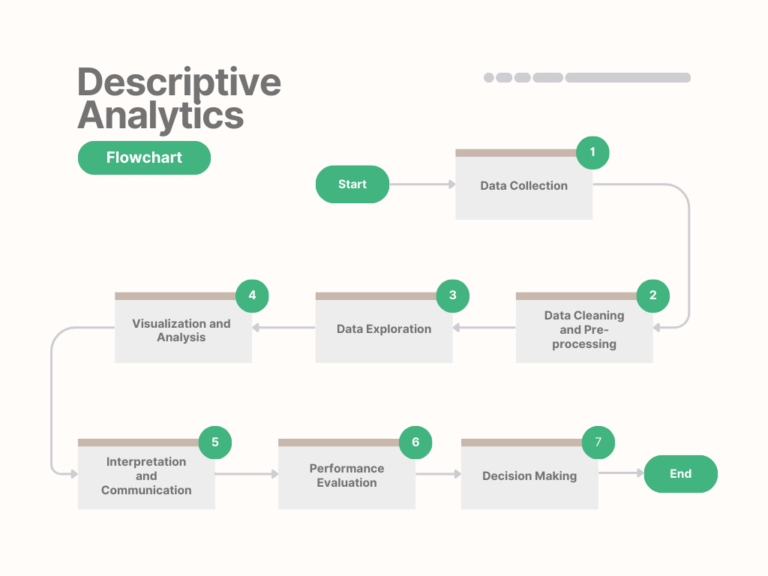
Diagnostic Analytics
In order to find insights, improve hypotheses, and comprehend results, diagnostic analytics is an iterative process that involves systematic and rigorous data study. Determining the issue or goal and compiling relevant historical data from a number of sources are the first steps in the process. To find trends and characteristics, exploratory data analysis (EDA), which is basically summarising, is carried out once the data has been pre-processed to guarantee quality. After this, hypotheses are developed and put to the test using statistical analysis and visualizations, which allows the detection or revelation of anomalies and causal connections. Contributing factors are found using root cause analysis, and the results are verified, analysed, and shared with relevant parties. Lastly, practical suggestions are offered to resolve problems, streamline procedures, or take advantage of possibilities.
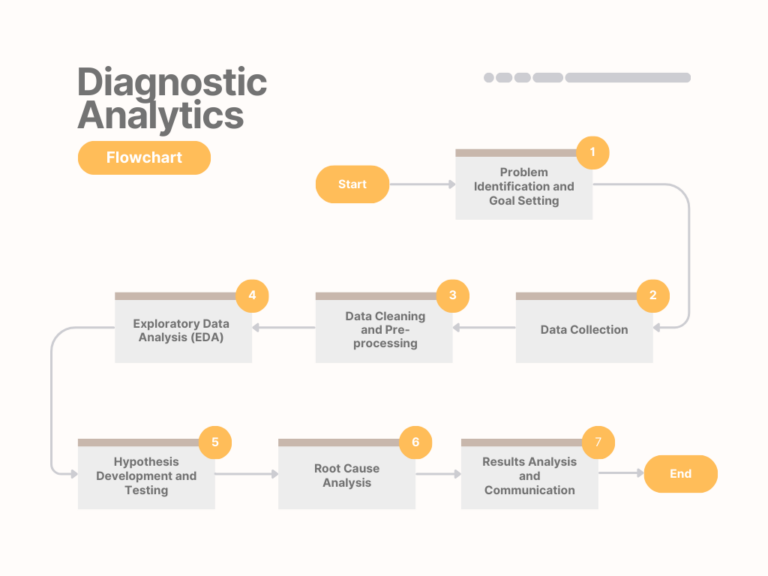
Predictive Analytics
Using historical data, predictive analytics develops models that analyses and forecasts future events. The accuracy of the models depends on the quality of data, model selection, and regular maintenance. To guarantee correctness, the process starts with collecting relevant data like input variables and required outcomes. After this, the data is cleaned and prepared for further steps. Next step is the feature selection and engineering which highlights essential factors. Once the features are selected, model selection is the next step which is one of the important steps which uses trains over historical data to learn patterns and trends. Hyperparameters adjustments is then used to evaluate and fine tune model’s performance. Once the model gets validated, the model is deployed with new data for it to make prediction and forecast new patterns. It then requires regular monitoring which make sures that accuracy is maintained with evolving patterns.
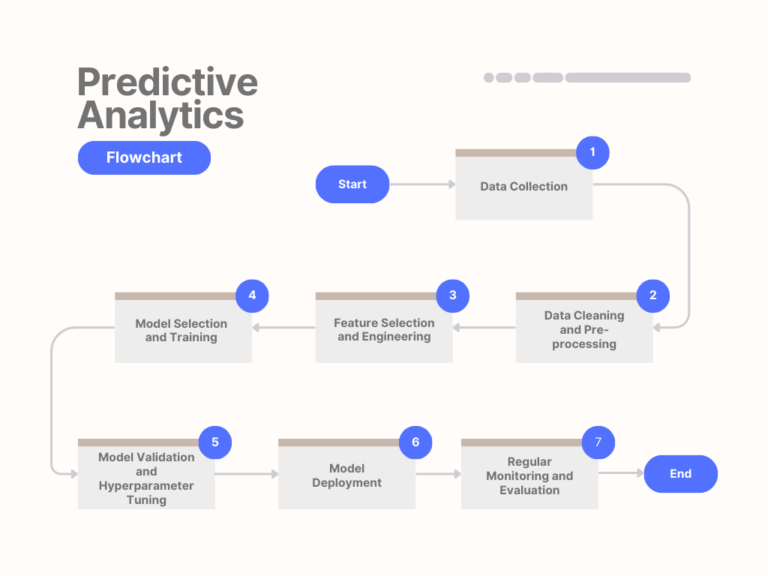
Prescriptive Analytics
Advanced techniques like predictive modelling, algorithms optimisation, and decision frameworks which optimally recommend actions; all these are used in prescriptive analytics. The collection and integration of data is the first step in the process. It is then followed by cleaning and making the data ready for further use. While prediction models just forecast what might happen in the future using the statistics and machine learning, whereas the prescriptive techniques identify the best course possible of action by taking an account of the contributing factors and data. The results from the prescriptive modelling are aligned with business rule and constraints which then yields results. Simulations are performed for various scenarios to explore effects of different decisions using multiple conditions. The basic plan is to get actionable insights which assist the decision makers to make practical decision which are beneficial for their businesses. After implementing, continuous monitoring and evaluation is necessary for maintaining accuracy and efficiency.
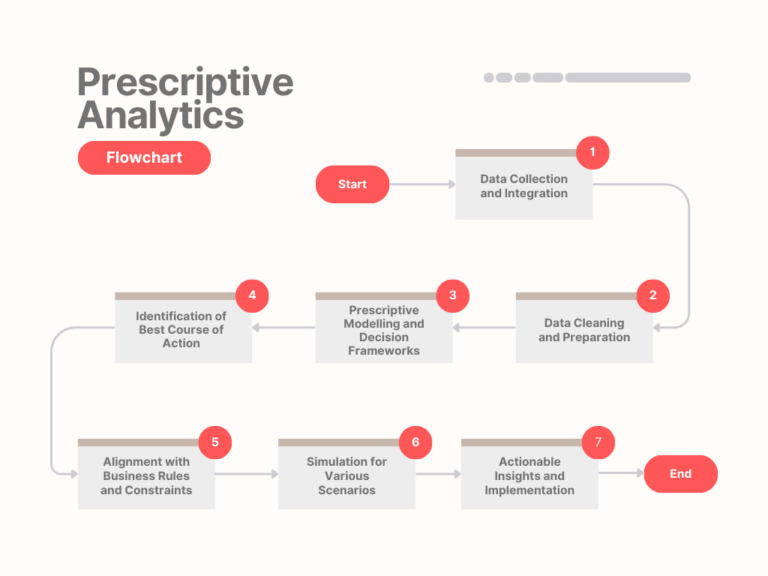
Conclusion
Data Analytics is more than just statistics. It opens new opportunities, assists innovations, and lead to sustainable growth. According to the needs of the organisation, utilising the capabilities of the various types of data analytics is the need of the hour. Assessing what needs to be done and accordingly choosing the right set of analytics makes the businesses flourish and give them a competitive edge over others. Data is something that will never stop to grow, so rather than just waiting for the results to come, using the data adequately and efficiently will benefits the businesses. Also, as the technology continuous to evolve, having a hands-on with the data analytics techniques will become increasingly vital.